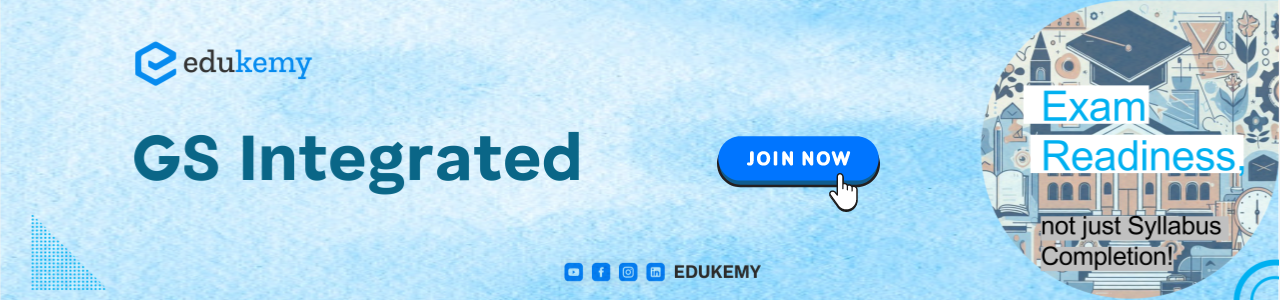
Big Data refers to the aggregation of “high-volume, high-velocity, or high-variety information assets that demand innovative processing methods to facilitate enhanced decision-making, insight discovery, and process optimization.” It represents vast datasets that conventional computing methods cannot effectively manage. The concept encompasses not only the data itself but also the diverse frameworks, tools, and methodologies employed in its analysis. Big Data comprises structured, semi-structured, and unstructured data amassed by organizations, utilized in machine learning initiatives, predictive modeling, and other sophisticated analytics endeavors. Big Data processing and storage systems, along with analytics tools, have become integral components of organizational data management infrastructures.
Contents
Mechanisms of Big Data
Data Collection
In the realm of data collection, each business adopts its unique strategy. Advancements in technology enable businesses to gather both structured and unstructured data from various sources such as cloud storage, mobile applications, in-store IoT sensors, among others.
Data Organization
Effective organization of data is imperative post-collection to ensure accurate responses to analytical inquiries, particularly when dealing with large and unstructured datasets.
Data Cleansing
Ensuring data quality is paramount for generating robust findings. Regardless of size, all data must undergo scrubbing to eliminate duplicate or redundant data while ensuring proper structuring. Dirty data can obscure insights and lead to erroneous conclusions.
Data Analysis
Transforming massive datasets into actionable insights requires time and advanced analytics techniques. Some of these techniques include:
- Data mining, which explores vast datasets to identify patterns, anomalies, and correlations.
- Predictive analytics, which leverages historical data to forecast future trends and potential risks.
- Deep learning algorithms, which unravel complex patterns within abstract data by simulating human learning patterns through layered algorithms.
Examples of Big Data
Big data encompasses a wide array of sources including transaction processing systems, customer databases, documents, emails, medical records, internet clickstream logs, mobile apps, and social networks. Machine-generated data, derived from network and server logs, as well as sensor data from manufacturing machines, industrial equipment, and IoT devices, also contribute to big data repositories. Moreover, big data environments often integrate external data on consumer behavior, financial markets, weather conditions, traffic patterns, geographic information, scientific research, among others.
Six V’s of Big Data:
Volume
Big Data denotes an extensive volume of information, with data size playing a pivotal role in determining its significance. The sheer volume of data is a defining characteristic of Big Data solutions, influencing their utility and complexity.
Variety
Variety encompasses the diverse array of data sources and formats, including structured and unstructured data. Unlike traditional sources limited to spreadsheets and databases, modern analysis considers data from emails, images, videos, sensors, and more. Managing this variety poses challenges in storage, mining, and analysis.
Velocity
Velocity denotes the speed at which data is generated and processed to meet demands. The potency of Big Data lies in its rapid generation and utilization. Big Data Velocity concerns the influx of data from various sources like business processes, social media, sensors, and mobile devices.
Variability
Variability reflects the inconsistency inherent in data, hindering effective management and processing. Dealing with the variability of data is crucial for ensuring accuracy and reliability in analytics processes.
Veracity
Veracity pertains to the reliability and accuracy of data sets. Raw data from diverse sources can introduce quality issues, leading to errors in analysis. Addressing veracity concerns involves robust data management and cleansing procedures to ensure the integrity of analytics outcomes.
Value
Value emphasizes the relevance and utility of collected data in addressing business needs. Not all data holds tangible business value, necessitating organizations to prioritize data relevance in Big Data analytics initiatives to derive meaningful insights and drive informed decision-making.
Applications of Big Data
- Enhanced Product Development
Companies like Netflix and Procter & Gamble utilize big data to forecast customer demand and develop predictive models for new products and services. By analyzing key characteristics and the commercial success of previous offerings, they can optimize product development processes.
- Predictive Maintenance
Predicting mechanical failures involves analyzing both structured data (e.g., equipment details) and unstructured data (e.g., sensor readings, error messages). Organizations can proactively deploy maintenance strategies, minimize downtime, and maximize equipment uptime by identifying potential issues before they escalate.
- Enhanced Customer Experience
Big data enables organizations to gain deeper insights into customer experiences by aggregating data from various sources such as social media, web visits, and call logs. This information helps improve customer interactions and deliver enhanced value.
- Fraud Detection and Compliance
Big data analytics aids in identifying patterns indicative of fraudulent activities and ensures compliance with evolving security regulations. By analyzing vast amounts of data, organizations can enhance fraud detection mechanisms and expedite reporting processes.
- Machine Learning Advancements
The availability of big data facilitates machine learning by providing extensive datasets for training models. This approach allows machines to learn from data patterns rather than relying solely on programmed instructions.
- Operational Efficiency
Big data analysis enables organizations to evaluate production processes, customer feedback, and returns to minimize disruptions and forecast future demands. By leveraging insights derived from big data, organizations can make informed decisions to enhance operational efficiency.
- Drive Innovation
Big data exploration uncovers interdependencies among various factors, enabling organizations to innovate and devise new applications. By analyzing trends, customer preferences, and market demands, organizations can drive innovation in product development and service delivery.
- Informed Financial and Planning Decisions
Big data insights empower organizations to make better financial and planning decisions by uncovering trends, patterns, and consumer behaviors. By leveraging data-driven insights, organizations can optimize resource allocation and strategic planning initiatives.
- Facilitate Data-Driven Decisions
Big data plays a pivotal role in revealing patterns and trends that inform data-driven decision-making processes. By harnessing the power of big data analytics, organizations can make informed decisions backed by empirical evidence and insights.
Government Actions & Strategies
- The NITI Aayog, in collaboration with private entities, is spearheading the development of the ‘National Data & Analytics Platform’. This platform aims to centralize sectoral data, providing citizens, policymakers, and researchers with a unified source of information.
- The ‘Big Data Management Policy’ formulated by the CAG represents a significant step forward in auditing large volumes of data generated by the public sector across states and union territories.
- The Ministry of Statistics and Programme Implementation has proposed the establishment of a ‘National Data Warehouse on Official Statistics’. Leveraging technology and big data analytics, this initiative seeks to enhance the quality of macro-economic aggregates.
- The adoption of Direct Benefit Transfer in schemes like MGNREGA and the authentication process through Aadhaar has been instrumental in curbing fraudulent beneficiaries, ensuring more targeted and effective welfare distribution.
- The Ministry of Agriculture has entered into a partnership with ISRO to utilize satellite technology for mapping agricultural assets, enhancing monitoring and management capabilities in the sector.
- Initiatives such as the Smart City Mission, Digital India, and digital economy proposals like the BHIM app are key government endeavors leveraging big data to foster improved governance and efficiency across various sectors in the country.
Challenges:
- Privacy Concerns: Big Data Analytics introduces significant challenges regarding digitization, particularly in terms of Data privacy and Net neutrality, which must be carefully addressed.
- Data Security: Incidents of Aadhaar data breaches underscore the critical need for the government to enhance the security and protection of the digital data it collects from citizens.
- Technical Hurdles: Big Data encounters inherent limitations such as:
- Inadequate infrastructure for data collection and management, Storage and computational constraints, Issues related to scalability and streaming.
- Governance Challenges: Effective policymaking using Big Data necessitates a consistent and dynamic approach from the government. Continuous evaluation of feedback and adaptable policy structures are crucial for ensuring benefits reach the grassroots level.
- Despite advancements in data storage technologies, data volumes continue to double every two years, posing significant challenges for organizations in managing and storing data effectively.
- Data usability depends on curation efforts. Cleaning and organizing data in a manner conducive to meaningful analysis requires substantial time and effort, with data scientists spending a majority of their time on these tasks.
- The proliferation of data variety, including semi-structured and unstructured data from sources like social media and the Internet of Things, presents a significant challenge. Traditional tools often struggle to efficiently process and analyze these diverse data types.
- Selecting the most suitable Big Data tool remains a challenge due to the multitude of options available. Choosing the wrong tool can result in wasted resources and inefficiencies.
- Data security emerges as a critical concern. Organizations, in their pursuit of data understanding and analysis, sometimes overlook data security, leaving unprotected data vulnerable to exploitation by hackers.
FAQs
Q: What is Big Data Technology?
Big Data Technology refers to the tools, techniques, and frameworks used to capture, store, manage, process, analyze, and visualize large volumes of structured and unstructured data to extract valuable insights and make data-driven decisions.
Q: What are the key components of Big Data Technology?
The key components of Big Data Technology include:
- Storage systems such as Hadoop Distributed File System (HDFS), NoSQL databases, and data warehouses.
- Processing frameworks like Apache Hadoop, Apache Spark, and Apache Flink.
- Data ingestion tools for collecting data from various sources.
- Data processing and querying languages such as SQL, HiveQL, and Pig Latin.
- Analytics and visualization tools for deriving insights from data, like Tableau, Power BI, and Apache Zeppelin.
Q: What are the benefits of using Big Data Technology?
Big Data Technology offers several benefits, including:
- Scalability: It allows organizations to handle large volumes of data efficiently.
- Real-time data processing: Enables organizations to analyze and act upon data in real-time.
- Cost-effectiveness: Open-source frameworks like Hadoop reduce the cost of storing and processing data.
- Improved decision-making: Provides insights from data analysis, leading to better decision-making processes.
- Competitive advantage: Enables businesses to gain insights into market trends, customer behavior, and operational efficiency, giving them a competitive edge.
Q: What are some common use cases of Big Data Technology?
Big Data Technology finds applications in various industries, including:
- E-commerce: Recommendation systems, personalized marketing, and customer behavior analysis.
- Healthcare: Predictive analytics for patient diagnosis, drug discovery, and personalized medicine.
- Finance: Fraud detection, risk management, algorithmic trading, and customer segmentation.
- Manufacturing: Predictive maintenance, supply chain optimization, and quality control.
- Social media: Sentiment analysis, user behavior analysis, and content optimization.
Q: What are the challenges associated with Big Data Technology?
Some challenges in Big Data Technology include:
- Data privacy and security concerns.
- Data integration from disparate sources.
- Scalability issues with growing data volumes.
- Complexity in selecting the right tools and technologies.
- Skill gap in terms of expertise in managing and analyzing big data.
In case you still have your doubts, contact us on 9811333901.
For UPSC Prelims Resources, Click here
For Daily Updates and Study Material:
Join our Telegram Channel – Edukemy for IAS
- 1. Learn through Videos – here
- 2. Be Exam Ready by Practicing Daily MCQs – here
- 3. Daily Newsletter – Get all your Current Affairs Covered – here
- 4. Mains Answer Writing Practice – here